Multiobjective Neural Architecture Search Challenge for Real-Time Semantic Segmentation
Competition News and Updates
June/26/2024: This competition is over, you can see the details of the competition on our Github. The ranking of the participating teams is as follows:
🥇 GrSMEA_NCHU
Authors: Chao He, Congxuan Zhang, Ming Li, Hao Chen, and Zige Wang
Affiliation: Nanchang Hangkong University, Nanchang, China
Description: Grid-Based Evolutionary Algorithm Assisted by Self-Organizing Map
🥈 MOEA-AP
Authors: Jiangtao Shen, Junchang Liu, Huachao Dong, Xinjing Wang, and Peng Wang
Affiliation: Northwestern Polytechnical University, Xi’an, China
Description: A Multiobjective Evolutionary Algorithm with Adaptive Simulated Binary Crossover and Pareto Front Modeling
🥉 IMS-LOMONAS
Authors: Quan Minh Phan and Ngoc Hoang Luong
Affiliations: University of Information Technology, Ho Chi Minh City, Vietnam; Vietnam National University, Ho Chi Minh City, Vietnam
Description: Pareto Local Search for Multi-objective Neural Architecture Search
4. DLEA
Authors: Gui Li and Guining Zhan
Affiliation: Huazhong University of Science and Technology, Wuhan, China
Description: Dynamic Learning Evolutionary Algorithm
5. IDEA_GNG
Authors: Bingsen Wang, Xiaofeng Han, Xiang Li, and Tao Chao
Affiliation: Harbin Institute of Technology, Harbin, China
Description: An improved Decomposition-based Multi-Objective Evolutionary Algorithm for Network Architecture Search
Apr/3/2024:The competition will be funded by the IEEE CIS, with awards of $500 USD for the first-place winner, $300 USD for the second-place winner, and $200 USD for the third-place winner.
Mar/12/2024(Important):EvoXBench has been updated to version 1.0.5. This change fixes the bugs of CitySeg/MOP10 and HV calculation. A detailed explanation of the problems has been added to the competition homepage, and the parameter setting section has been updated. To avoid any unforeseen issues, we strongly recommend that all participants and users utilize the latest version of EvoXBench(i.e., pip install evoxbench==1.0.5).
Background
The field of Neural Architecture Search (NAS) has undergone significant advancements, attracting considerable interest, especially in optimizing for multiple objectives simultaneously. NAS focuses on automating the design of artificial neural networks, aiming to discover architectures that can perform exceptionally well on specific tasks.
A critical application of advanced NAS is in real-time semantic segmentation, particularly pivotal in autonomous driving systems. Real-Time semantic segmentation involves the process of instantaneously classifying each pixel in an image into various categories, which is crucial for autonomous vehicles to navigate safely. This involves not only recognizing objects like cars, pedestrians, and road signs but also understanding the context of each element in the environment. Accurate and fast semantic segmentation allows for immediate decision-making, a non-negotiable requirement in autonomous driving where delayed responses can lead to critical consequences.
The evolution from the previous multiobjective NAS competition at IEEE CEC 2023, which focused on image classification, to the current challenge in real-time semantic segmentation marks a significant and strategic shift in the field. The previous competition highlighted the effectiveness of evolutionary multiobjective optimization (EMO) algorithms in balancing performance with computational efficiency in NAS for image classification. This progress has set a robust foundation for venturing into more complex realms like real-time semantic segmentation, a domain with higher stakes and more demanding requirements. This transition reflects the growing need for sophisticated NAS solutions capable of real-time processing in critical applications such as autonomous driving. It emphasizes the urgency for NAS systems that are not just accurate, but also exceptionally fast and reliable, catering to the real-world, time-sensitive demands of scenarios like autonomous vehicle navigation.
Task
Building on the success of the 2023 Multiobjective Neural Architecture Search Competition, the 2024 edition introduces a new set of challenges focused on the semantic segmentation of urban landscapes, particularly on the Cityscapes dataset. This year’s competition, while retaining the core essence of using EMO algorithms for NAS, shifts its focus towards more complex and realistic scenarios encountered in semantic segmentation tasks.
Participants are required to utilize EvoXBench, an end-to-end pipeline that has been meticulously updated to incorporate the new challenges of this year’s competition. EvoXBench facilitates the efficient evaluation of EMO algorithms without the necessity of GPUs or Pytorch/Tensorflow. It provides a comprehensive environment encompassing various search spaces, architectural types, datasets, hardware specifications, and optimization objectives.
The task for this year’s competition revolves around 15 uniquely tailored instances within the CitySeg/MOP segment of EvoXBench, each designed for semantic segmentation on the Cityscapes dataset. As summarized in Table I, these instances range in complexity, with the number of optimization objectives varying from two to seven per instance. The objectives cover a range of key performance metrics: \( f^e \) represents the model segmentation error; \( f^c \) encompasses model complexity parameters, including the number of floating-point operations (FLOPs, denoted as \( f_1^c \)) and the count of model parameters or weights (\( f_2^c \)).Additionally,\( f^{\mathcal {H}} \) addresses hardware device performance, capturing critical aspects such as latency (\( f_1^h \), the reference latency on the given hardware) and energy consumption (\( f_2^h \), measured in Joules on the corresponding hardware). These hardware-related objectives include \( h_1 \) for GPU performance and \( h_2 \) for edge device efficiency, thereby encompassing a wide spectrum of real-world application scenarios.
TABLE I: Definition of the CitySeg/MOP test suite.
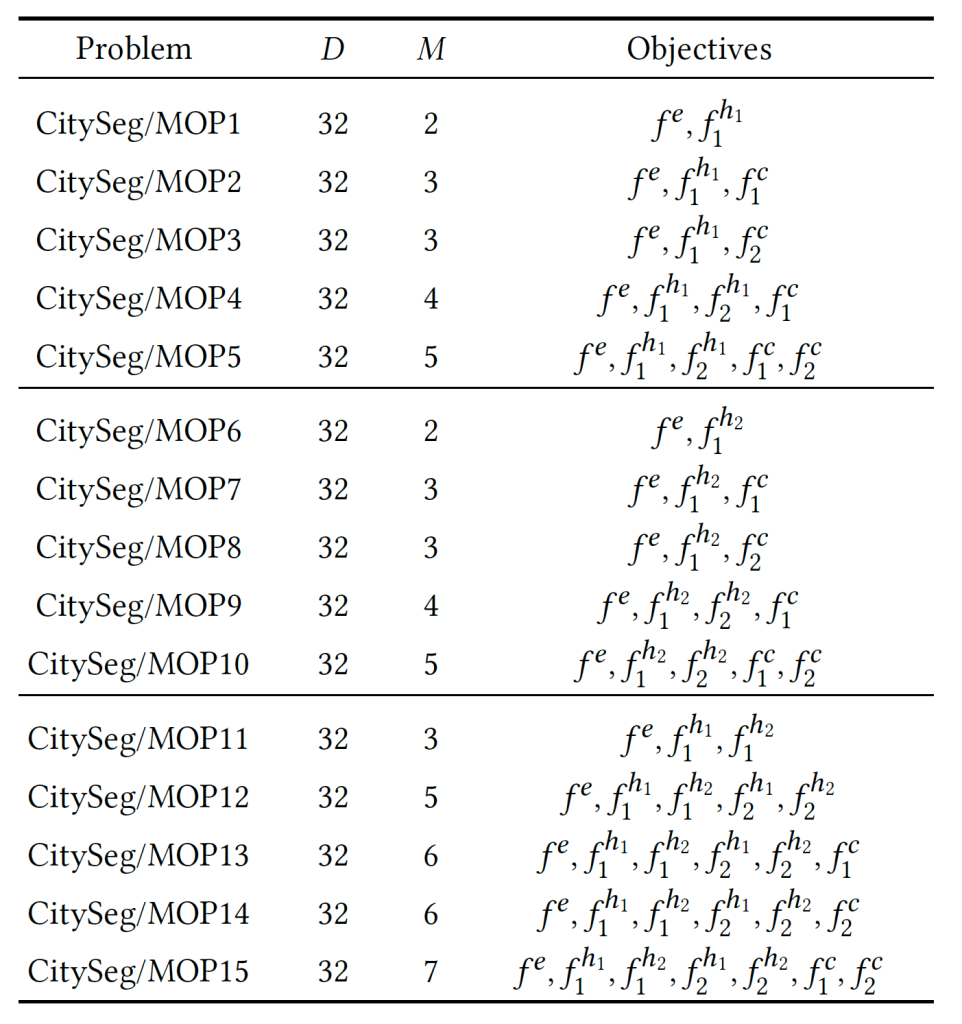
Note:
The 24-bit or 32-bit encoding length is a different representation of the same encoding format.
For ease of understanding, the encoding length is described as 32 bits long in illustrative documents, and consists of the following diagrams.
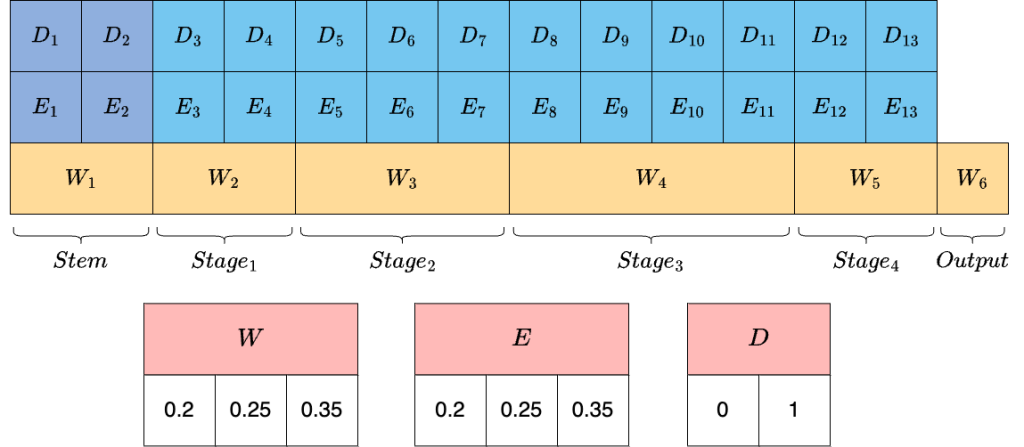
The value of D is 0 or 1, which indicates whether there is a neural network layer here.
In the code, for the convenience of encoding and decoding of the network architecture, the encoding of D is simplified to describe the number of layers in stem and each stages. So for this encoding, its length contains 5 encoding bits describing D, and the total length is 5+13+6=24.
2. Parameter settings
The population size of each problems is set in Table II. The algorithm used required 31 independent runs using 10,000 fitness evaluations.
TABLE II: Parameter settings.

Tutorials
1. To better understand EvoXBench and the formulas of NAS problems, you can browse this paper [1] and watch the tutorial video.
Tutorial materials can be downloaded from: tutorial_materials.zip
2. Install EvoXBench. The installation tutorial refers to the project site of EvoXBench.
3. Run the example code, you can familiarize yourself with how to use EvoXBench. [Python] [Mathlab] [Java]
4. Develop your own EMO algorithm and calculate the indicators with the provided performance indicator tool to check the performance of the algorithm.
For MATLAB users, we recommend using the PlatEMO platform to develop algorithms. The benchmark test suites in EvoXBench have been embedded in PlatEMO, you only need to read the examples to know how to use it. Before running PlatEMO, you need to run “evoxbenchrpc” in the terminal. Then run the MATLAB code of your algorithm.
Evaluation Criteria
In this competition, we use Hypervolume (HV) as performance metrics. During the evaluation, EvoXBench will be used to evaluate “X” and calculate the HV. All submitted results will be used to calculate HV in python on the same computer.
Submission
Please submit the compressed folder (a .zip file) of your algorithm and send it to zhenyuliang97@gmail.com no later than May 31, 2024.
The .zip file should contain a report, algorithm source code and 15 result files.
The report must include the following sections: (i) title; (ii) names, affiliations, and emails of participants; (iii) description of the algorithm; (iv) mean and standard deviation of HV values from 31 runs per test instance.
The result of each instance should be saved in json format (i.e.,15 .json file). The list of dictionaries is stored in the .json file.
The structure of the .json file is as follows:
[{“run”:1, “X”: [[0, 1, 2, …], [0, 0, 1, …], …, [1, 1, 1, …]], “F”: [[0.19, 39606472], [0.24, 7247656], …, [0.23, 7531312]], “HV”: 0.9},
{“run”: 2, “X”: [[0, 1, 2, …], [0, 0, 1, …], …, [1, 1, 1, …]], “F”: [[0.19, 39606472], [0.24, 7247656], …, [0.23, 7531312]], “HV”: 0.9},
…,
{“run”: 31, “X”: [[0, 1, 2, …], [0, 0, 1, …], …, [1, 1, 1, …]], “F”: [[0.19, 39606472], [0.24, 7247656], …, [0.23, 7531312]]}, “HV”: 0.9}]
where “run” is the sequence number of each run test instance; “X” denotes an encoded network architecture (i.e., decision vector); “F” is the objective function on validation mode; “HV” is performance metric.
Awards
The competition will award the top three participants.
Questions
If you have any question to the competition , please contact Zhenyu Liang(zhenyuliang97@gmail.com)and Yifan Zhao(AlpAcA0072@gmail.com) or join the QQ group to ask questions (Group number: 297969717).
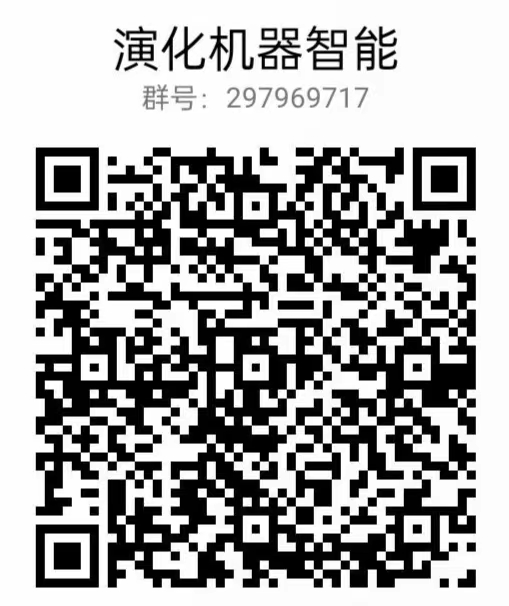
Organizers
Name: Zhenyu Liang
Affiliation: Department of Computer Science and Engineering, Southern University of Science and Technology, Shenzhen, China. (Email: zhenyuliang97@gmail.com)
Short Bio: Zhenyu Liang received the B.Sc. from Southern University of Science and Technology in 2020. He is currently a master student in the Department of Computer Science and Engineering, Southern University of Science and Technology. His main research interests are evolutionary algorithms and evolutionary multi- and many-objective optimization. Zhenyu Liang serves as the Chairs of Social Media of IEEE Computational Intelligence Society (CIS) Shenzhen Chapter.
Name: Yifan Zhao
Affiliation: Department of Computer Science and Engineering, Southern University of Science and Technology, Shenzhen, China. (Email: AlpAcA0072@gmail.com)
Short Bio: Yifan Zhao received the B.Sc. from Southern University of Science and Technology in 2022. He is currently a master student in the Department of Computer Science and Engineering, Southern University of Science and Technology. His main research interests are evolutionary algorithms, multiobjective optimization and neural architecture search.
Name: Zhichao Lu
Affiliation: Department of Computer Science, City University of Hong Kong, HK. (Email: luzhichaocn@gmail.com)
Short Bio: Zhichao Lu is currently an Assistant Professor in the Department of Computer Science, City University of Hong Kong. He received his Ph.D. degree in Electrical and Computer Engineering from Michigan State University, USA, in 2020. His current research focuses on the intersections of evolutionary computation, learning, and optimization, notably on developing efficient, reliable, and automated machine learning algorithms and systems, with the overarching goal of making AI accessible to everyone.
Dr Zhichao Lu serves as the Co-Chair of Member Activities of the IEEE Computational Intelligence Society (CIS) Shenzhen Chapter, and Vice Chair of the IEEE CIS Task Force on Intelligent Systems for Health. He is the co-leading organizer of CVPR 23 Tutorial on Evolutionary Multi-Objective for Machine Learning. He is the recipient of the GECCO Best Paper Award (2019) and the SUSTech Presidential Outstanding Postdoctoral Award (2020).
Name: Ran Cheng
Affiliation: Department of Computer Science and Engineering, Southern University of Science and Technology, Shenzhen, China. (Email: ranchengcn@gmail.com)
Short Bio: Ran Cheng is currently an Associate Professor with the Southern University of Science and Technology (SUSTech), China. He received the PhD degree in computer science
from the University of Surrey, UK, in 2016. His primary research interests lie at the intersection of evolutionary computation and neural computation, aimed at delivering high-performance computational solutions that address optimization and modeling challenges in contemporary science and engineering.
Dr Ran Cheng has published over 100 peer-reviewed papers, with over 10,000 Google Scholar citations. He is the founding Chair of IEEE Computational Intelligence Society (CIS) Shenzhen Chapter. He serves as an Associate Editor for several journals, including: IEEE Transactions on Evolutionary Computation, IEEE Transactions on Emerging Topics in Computational Intelligence, IEEE Transactions on Cognitive and Developmental Systems, IEEE Transactions on Artificial Intelligence, ACM Transactions on Evolutionary Learning and Optimization, etc. He is the recipient of the IEEE Transactions on Evolutionary Computation Outstanding Paper Awards (2018, 2021), the IEEE CIS Outstanding PhD Dissertation Award (2019), the IEEE Computational Intelligence Magazine Outstanding Paper Award (2020). Dr Ran Cheng has been featured as among the World’s Top 2% Scientists (2021 – 2023) and the Clarivate Highly Cited Researchers (2023). He is a Senior Member of IEEE.
References
[1] Z. Lu, R. Cheng, Y. Jin, K. C. Tan and K. Deb, “Neural Architecture Search as Multiobjective Optimization Benchmarks: Problem Formulation and Performance Assessment,” in IEEE Transactions on Evolutionary Computation, doi: 10.1109/TEVC.2022.3233364.
[2] Z. Lu, R. Cheng, S. Huang, H. Zhang, C. Qiu and F. Yang, “Surrogate-Assisted Multiobjective Neural Architecture Search for Real-Time Semantic Segmentation,” in IEEE Transactions on Artificial Intelligence, vol. 4, no. 6, pp. 1602-1615, Dec. 2023, doi: 10.1109/TAI.2022.3213532.