Hui Bai, Ran Cheng*, Danial Yazdani, Kay Chen Tan, Yaochu Jin
Abstract:
Variable grouping provides an efficient approach to large-scale optimization, and multipopulation strategies are effective for both large-scale optimization and dynamic optimization. However, variable grouping is not well studied in large-scale dynamic optimization when cooperating with multipopulation strategies. Specifically, when the numbers/sizes of the variable subcomponents are large, the performance of the algorithms will be substantially degraded. To address this issue, we propose a bilevel variable grouping (BLVG)-based framework. First, the primary grouping applies a state-of-the-art variable grouping method based on variable interaction analysis to group the variables into subcomponents. Second, the secondary grouping further groups the subcomponents into variable cells, that is, combination variable cells and decomposition variable cells. We then tailor a multipopulation strategy to process the two types of variable cells efficiently in a cooperative coevolutionary (CC) way. As indicated by the empirical study on large-scale dynamic optimization problems (DOPs) of up to 300 dimensions, the proposed framework outperforms several state-of-the-art frameworks for large-scale dynamic optimization. [Source Code]
Results
Overall Comparison
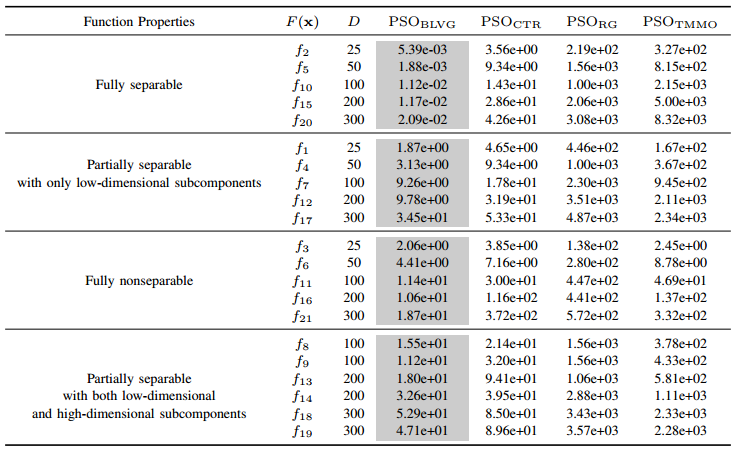
Influence of Different Optimizers
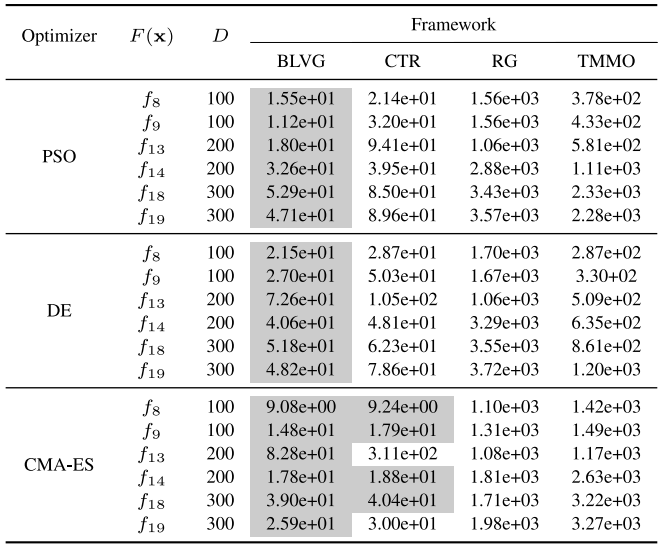
Influence of Different Variable Grouping Methods
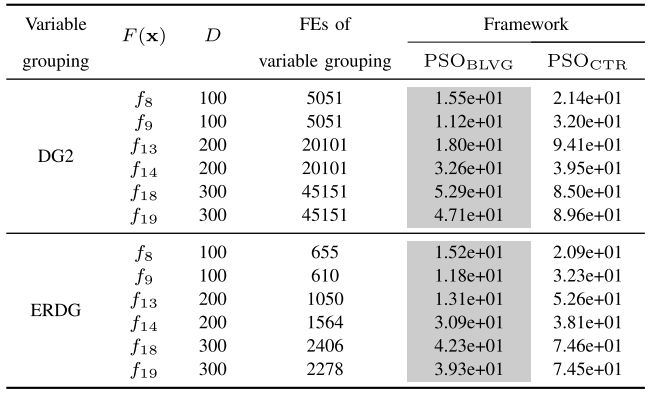
Robustness to Dynamic Changes
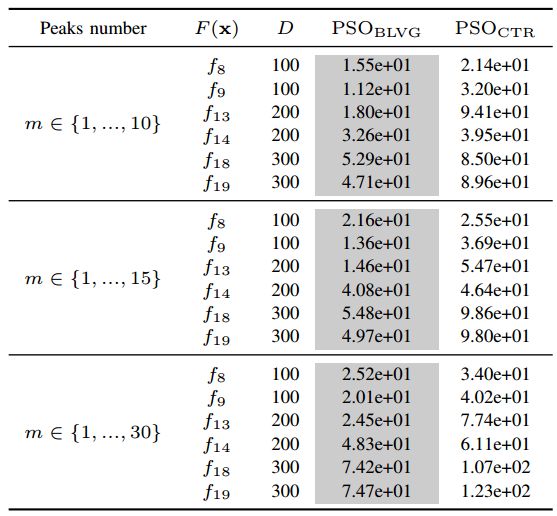
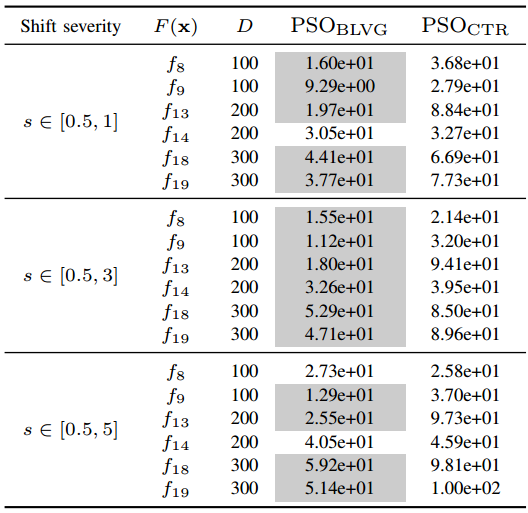
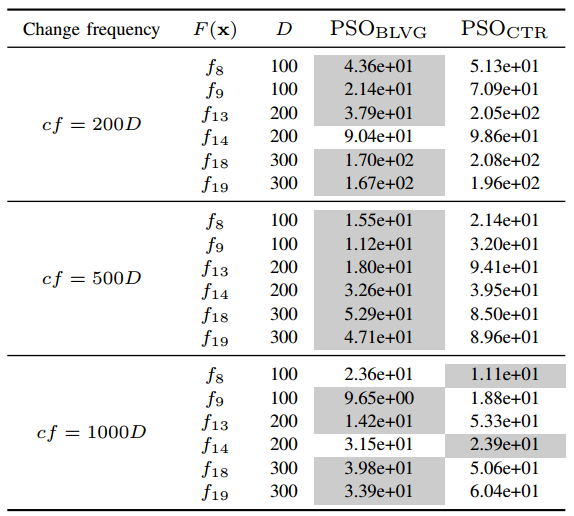
Citation
@ARTICLE{9772492,
author={Bai, Hui and Cheng, Ran and Yazdani, Danial and Tan, Kay Chen and Jin, Yaochu},
journal={IEEE Transactions on Cybernetics},
title={Evolutionary Large-Scale Dynamic Optimization Using Bilevel Variable Grouping},
year={2022},
volume={},
number={},
pages={1-14},
doi={10.1109/TCYB.2022.3164143}}
Acknowledgments:
This work was supported in part by the National Natural Science Foundation of China under Grant 61906081; in part by the Shenzhen Science and Technology Program under Grant RCBS20200714114817264; in part by the Guangdong Provincial Key Laboratory under Grant 2020B121201001; and in part by the Program for Guangdong Introducing Innovative and Entrepreneurial Teams under Grant 2017ZT07X386.